Review - RAID | Benchmark for machine-generated text review
RAID Benchmark for machine-generated text review
I am by no means an expert in machine learning, and especially in detecting machine-generated text, but it’s fun to do a review, so here it is. And maybe some things I found interesting not having that background.
Some background
Machine generation detection is basically stating if a piece of text is generated by an llm (like ChatGPT) versus a human writing it. This is super important nowadays. For example, people doubt if others are bots on online platforms and if they’re just talking to ChatGPT. And if you can’t, well… that means AGI???
Into the paper
Yeah I just mostly look at graphs/charts, cause it’s pretty.
The papers goes through generating a set of data with human/machine generated text (on different topics ~ news, reddit, poetry, wikipedia, etc) with different models (GPT 2/3/4, mistral, etc) and then evaluates the detectors on the dataset to see if the detectors are actually good.
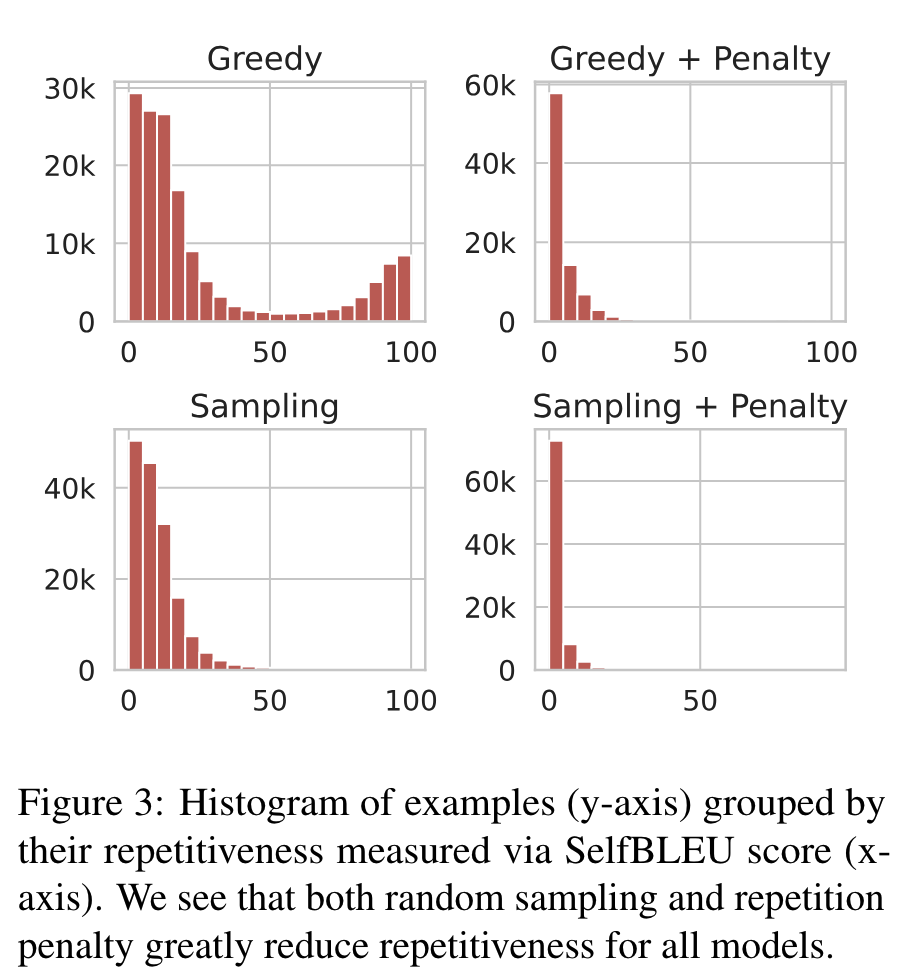
This is pretty cool. It seems to be obvious that ramdom sampling will decrease SelfBLEU, but it’s nice to see the numbers.
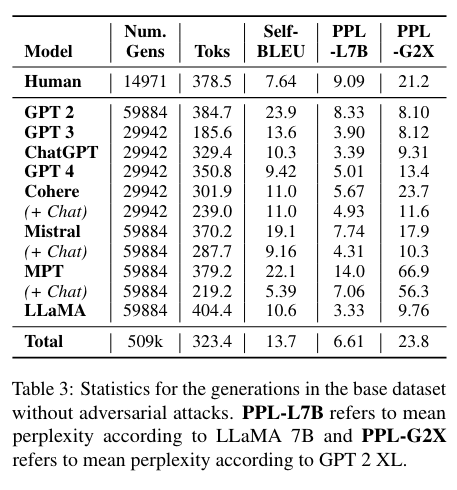
Some questions about fig 4. Seems like the generation of GPT3/4/ChatGPT/Cohere are 1/2 of the others (probably cost). Another thing is the MPT+Chat Self-BLEU which is even lower than human (5.39 vs 7.64). This is pretty cool and is shown in the text itself. But how is done and does this matter?
Topic | Title | Human | MPT+Chat | GPT4 |
---|---|---|---|---|
abstracts | FUTURE-AI: Guiding Principles and Consensus Recommendations for Trustworthy Artificial Intelligence in Future Medical Imaging | The recent advancements in artificial intelligence (AI) combined with the extensive amount of data generated by today’s clinical systems, has led to the development of imaging AI solutions across the whole value chain of medical imaging, including image reconstruction, medical image segmentation, image-based diagnosis and treatment planning. Notwithstanding the successes and future potential of AI in medical imaging, many stakeholders are concerned of the potential risks and ethical implications of imaging AI solutions, which are perceived as complex, opaque, and difficult to comprehend, utilise, and trust in critical clinical applications. Despite these concerns and risks, there are currently no concrete guidelines and best practices for guiding future AI developments in medical imaging towards increased trust, safety and adoption. To bridge this gap, this paper introduces a careful selection of guiding principles drawn from the accumulated experiences, consensus, and best practices from five large European projects on AI in Health Imaging. These guiding principles are named FUTURE-AI and its building blocks consist of (i) Fairness, (ii) Universality, (iii) Traceability, (iv) Usability, (v) Robustness and (vi) Explainability. In a step- by-step approach, these guidelines are further translated into a framework of concrete recommendations for specifying, developing, evaluating, and deploying technically, clinically and ethically trustworthy AI solutions into clinical practice. | The FUTURE-AI study presents guiding principles and consensus recommendations aimed at ensuring trustworthy artificial intelligence (AI) applications in future medical imaging. The document outlines seven focus areas, including human values & ethics; transparency/interpretability of AI models; training data quality management; validation testing procedures; performance monitoring protocols; governance frameworks that regulate responsible innovation | This paper presents a consensus among experts for shaping the landscape of trustworthy Artificial Intelligence (AI) in future medical imaging. This study, conducted by FUTURE-AI, identifies a set of guiding principles and provides specific and actionable recommendations for deploying AI in medical imaging. The guidelines center on three key pillars: fairness, transparency, and ethical considerations. The study also explores potential risks and challenges involved, including data security and privacy, algorithmic bias, and the need for robust AI models. These consensually agreed principles and recommendations serve as a roadmap to ensure that AI developments in medical imaging not only advance clinical care but are also safe, efficient, and fair. This approach contributes towards a more responsible and conscientious application of AI in healthcare. |
350hrs in and I still have no idea what I’m doing | So, I’ve been playing the game on and off for the past 3 years and I still feel like a noob. Something that I really struggle with is combat. I just spent my entire weekend putting together a $185 million fer-de-lance build. The moment I entered the battlefield I was destroyed. I thought the FDL was meant to have insane shielding and maneuverability but my shields were gone in 30 seconds and I was outmaneuvered by a python. My hull was destroyed in about 10 seconds. Even with 4 seeking missiles I only dealt 30% to the python. WTF. Can anyone tell me why this happens and how to get better in the future? How do I find what weapons are good for me? How do I survive longer than 1 minute in a low intensity conflict zone? | I’m currently playing BDO, and while it’s an amazing game with so much to explore and do, sometimes I feel like I’m just treading water and don’t know if I’m really making any progress… It can be overwhelming trying to figure out which skills to prioritize or how to earn the most silver, but at the same time, that’s part of the challenge and fun! Anyone else feeling this way? | Is this normal or am I just a slow learner? I mean, I enjoy the game and I absolutely love the world building and visuals. The mysteries and side quests are thrilling as well. But even after logging in over 350 hours into the game, I am still saying, “Wait, what?” every few minutes. I’m having trouble working out the core gameplay mechanics and wrapping my head around the subtleties of the combat. This is especially frustrating when you get into multiplayer mode where other players seem to be breezing through. The numerous characters and their hundreds of abilities and skill trees are just mind-boggling. I can’t seem to make a competent build that works well in all scenarios, and I still haven’t figured out the optimal equipment or weapon for each objective or enemy type. At first, I thought the game wasn’t very new user friendly, but seeing comments from other players about how they “got the hang of it” within the first 100 hours or so, I’m beginning to question my gaming skills. I’ve been playing games for years, and I’ve never felt so lost before. Either there’s a steep learning curve that I’m yet to get past, or I’m just not very good at this game. Nonetheless, I’m still enjoying it even though I’m blundering around like a headless chicken. Does anyone else feel the same or do I just need to spend more time getting into the finer details of the game? Any tips that might help me better understand the game mechanics and strategy would be much appreciated. Or should I just relax and enjoy the chaotic journey? | |
wiki | History of Madagascar | The history of Madagascar is distinguished clearly by the early isolation of the landmass from the ancient supercontinent containing Africa and India, and by the island’s late colonization by human settlers from the Sunda islands (Malay Archipelago) and from East Africa. These two factors facilitated the evolution and survival of thousands of endemic plant and animal species, some of which have gone extinct or are currently threatened with extinction due to the government not allocating resources to help the growing population, causing many people to resort to harmful environmental practices as a way of survival. Trade in the Indian Ocean at the time of first colonization of Madagascar was dominated by large ships, called Djong, coming from Java and Sumatra. Over the past two thousand years the island has received waves of settlers of diverse origins including Austronesian, Bantu, Arab, South Asian, Chinese and European. The majority of the population of Madagascar today is a mixture of Austronesian and Bantu settlers. Despite popular belief, there has been no genetic input from Arabs or Indians, although one tribe, the Antemoro, claims descent from Somali Arab traders. European and Middle eastern paternal ancestry is also present. | Madagascar is an island country located in the Indian Ocean, off the coast of Southeast Africa. The history of Madagascar can be traced back to its earliest human settlements around 1,200 BCE.[1] Over time, various kingdoms and states emerged on the island including the Vazimba Kingdom which was known for their military prowess[2]. In the 16th century, the first Europeans arrived at Madagascar with Portuguese explorer Diego Dias being the first recorded European visitor [3]. However, it wasn’t until the French colonization period that Western influence began to greatly impact daily life. In 1883 Prime Minister Rainilaiarinina established autonomy from France, although full independence would not come till 1960 when Malgache Republic gained recognition as one of four French Community members (alongside Algeria Metropolitan territory and overseas departments) under Constitution-of-1946 style framework. This lasted only briefly however before General Charles de Gaulle’s repeal made all but metropolitan territories fully autonomous; afterwards President Philibert Tsiranana requested membership within Union française like other African countries had been granted previously though this too failed attaining support primarily due political pressure exerted upon participants deliberating matters during 1958 Brussels Conference where issue first came up publicly while also marking heightened intensity decolonization efforts happening across Continent altogether that same year. With no option left behind closed doors amidst increasing international isolation resulting from continued application colonial policies even long after others declared themselves independent nations openly recognizable outside Europe itself through diplomatic representatives recognized worldwide according UN member status maintained formally recognised embassy level representation), leaders finally agreed negotiate terms compromise recognising sovereignty sovereign equal standing alongside former colony Metropole retaining limited authority over certain administrative functions such defence foreign affairs wherein executive arm central government responsible handling remainder issues pertaining internal administration including economic policy making decisions related trade commerce agreements concluded behalf entire nation rather than individual territorial subdivisions comprised federation structure originally proposed alternative solution put forth earlier discussions preceding official referendum held between September 1961 December 1962 resulted final decision confirming wish preserve close links remaining intact despite now formal separation complete legal effect February 6, 1959 marks date commemoration celebrated yearly anniversary achieving independencely hosted annual parade festivities presided ceremonial review troops usually headed highest ranking officer serving active duty position Chief Staff Special Operations Command Unit positioned headquarters Tana Capitol district capital city Antananarivo commonly referred locals abbreviated form TANA given common shorthand designation used | The history of Madagascar has been marked by the influence of numerous people and cultures from across the Indian Ocean, European and Arab traders, as well as indigenous groups. This diversity is reflected in the rich cultural heritage and linguistic variety found on the island today. Early Settlement and Austronesian Influence The first settlers of Madagascar were most probably Austronesians from Borneo, who arrived between 350 BC and 550 AD. These early settlers developed a unique culture and agriculture system, with the primary crop being rice, which still remains the staple food of Madagascar. They also built impressively constructed tombs and burial sites, some of which are still used today. Bantu Migration and Arab Influence Between 1000 and 1500 AD, Bantu- speaking Africans from the Mozambique channel also arrived on the island. This group introduced cattle farming, iron and metalworking skills to the island. Meanwhile, Arab traders who came to Madagascar during this period introduced Islam and the Arabic script. European Colonization The Portuguese were the first Europeans to discover Madagascar in 1500. Later French, British and Dutch traders also arrived. This contact led to the formation of several small kingdoms on the island. However, the Merina Kingdom, which was established in the central highlands in 1820, managed to unite most of these kingdoms under one rule. In 1896, France colonized Madagascar and introduced French language, educational system, and other aspects of French culture. The colonial period was marked by many violent uprisings, including the 1947 Malagasy Uprising, one of the first significant revolts against French colonial rule. Independence and Post-colonial Era Madagascar gained independence from France in 1960. The country’s post-colonial period has been marked by political instability, with numerous coups and changes in government. Despite the political challenges, Madagascar has made progress in education, public health, and economic development. However, economic disparities and environmental challenges, such as deforestation and soil erosion, remain significant issues. Madagascar’s history, richly influenced by people and cultures from both in and out of Africa, has shaped a unique cultural and biological diversity that is globally recognized today. Despite facing many challenges, the country continues to strive for progress and development. To date, research into Madagascar’s history is ongoing, adding more layers and understanding to this multicultural island’s fascinating past. The island’s history still shapes its present and will undoubtedly influence its future in the years to come |
^ All machine generated text is using sampling
On a side side, it’s interesting to see how different the generations are based on the model. The closed source models’ generation seem to be pretty good (overall). Open source seems to repeat quite often.
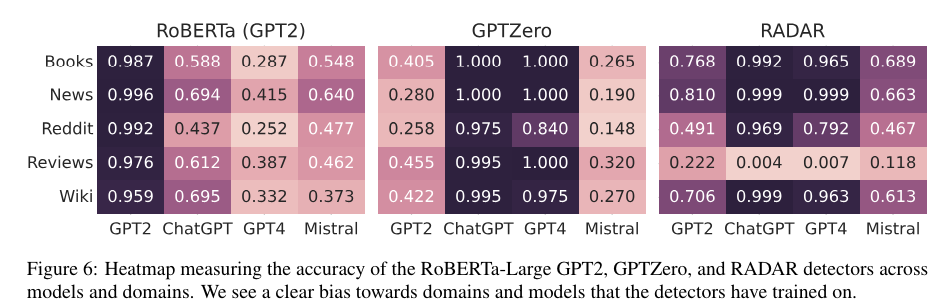
Cool detection of the domains the detectors were trained on. Seemed to be applied to figuring out what models are trained on (like what sources GPT4 were trained on). Applies to closed source programs, in context of security aspect (programs generally of are X format based on the author’s experience).
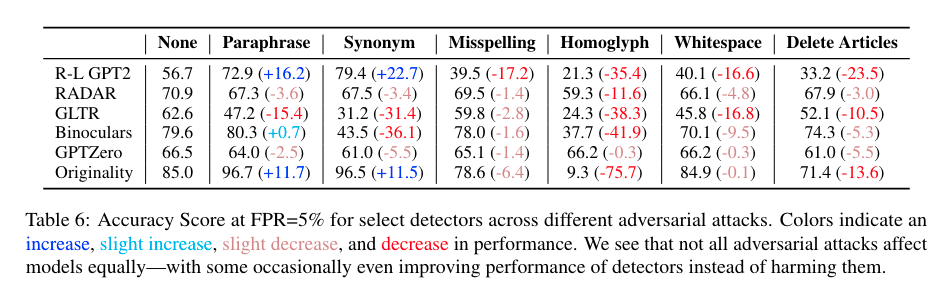
Intesting table. To create a best version of this, you can combine each model for each type of attack (if you can classify which attack it is). Another idea is to train a classifier to check if generated text has an attack and then use a good model to verify if it is an actual attack. I have no idea if this actually works.
It seems that homoglyph and whitespace and deleted aricles seem to affect the detectors the most. I wonder why. Is it because replacing/removing critical words/phrases causes the distribution of inputs tokens to be “out of bounds” of sorts. It would be interesting to see what the model layers are showing.
Here are the definitions of homoglyph, whitespace and deleted articles
Article Deletion - We search through the text and find every instance of the articles “a”, “an”, and “the”. We then randomly sample a fixed percentage θ of the possible mutations to make with a set seed and apply the attack at those indices.
Homoglyph - Homoglyphs are character that are non-standard unicode characters that strongly resemble standard English letters. These are typically characters used in Cyrillic scripts. We use the set of homoglyphs from Wolff (2020) which includes substitutions for the following standard ASCII characters: a, A, B, e, E, c, p, K, O, P, M, H, T, X, C, y, o, x, I, i, N, and Z. We limit ourselves to only homoglyphs that are undetectable to the untrained human eye, thus we are able to use an attack rate of θ = 100% and apply the attack on every possible character. For characters that have multiple possible homoglyphs we randomly choose between the homoglyphs.
Whitespace - This attack randomly selects some θ percent of inter-token spaces and adds an extra space character inbetween the tokens. This can occasionally result in multiple spaces added between two tokens as sampling is done with replacement.
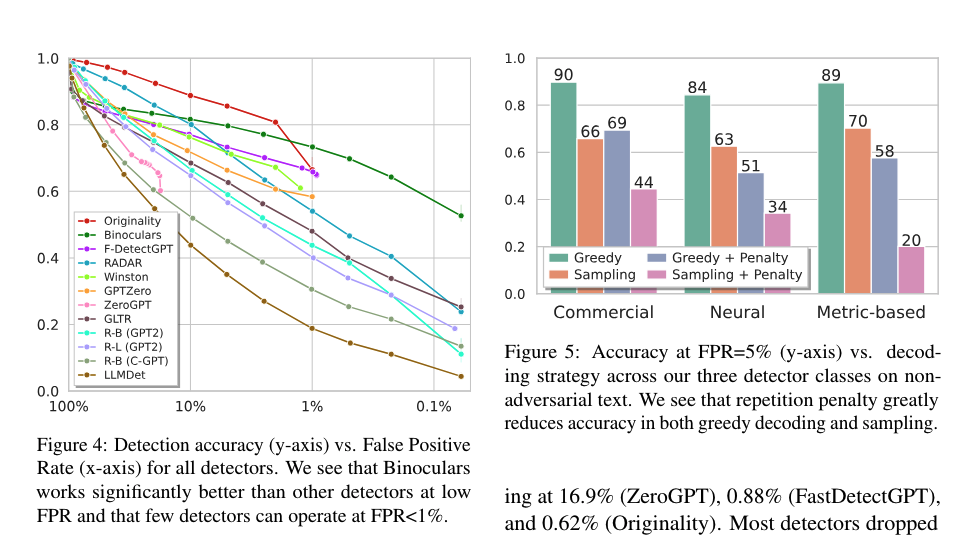
FPR (False positive rate) - “In practical terms, accuracy at a fixed FPR of 5% represents how well each detector identifies machine-generated text while only misclassifying 5% of human-written text”. Binoculars is a clear winner overall. I’m curious as to why and what techniques. Also, Binoculars does not do well in some domains.
Figure 5 shows something even more interesting. That with Sampling + Penalty, the accuracy drops the most while greedy is best. This shows the reverse of the SelfBLEUxX score (more repetitive). So is there a tradeoff between accuracy and SelfBLEU?
Appendix gem
Actually wtf, the best results are shown in the appendix…
It defines all the models, the attacks, the dataset. No reference. I should have read to this part first. I don’t have a strong background on any of it and this is a great mine of knowledge.
Some things I learned about more on detail on my own:
Datasets - CommonCrawl, multilingual Wikipedia, f deduplicated C4, the RedPajama10 split of CommonCrawl
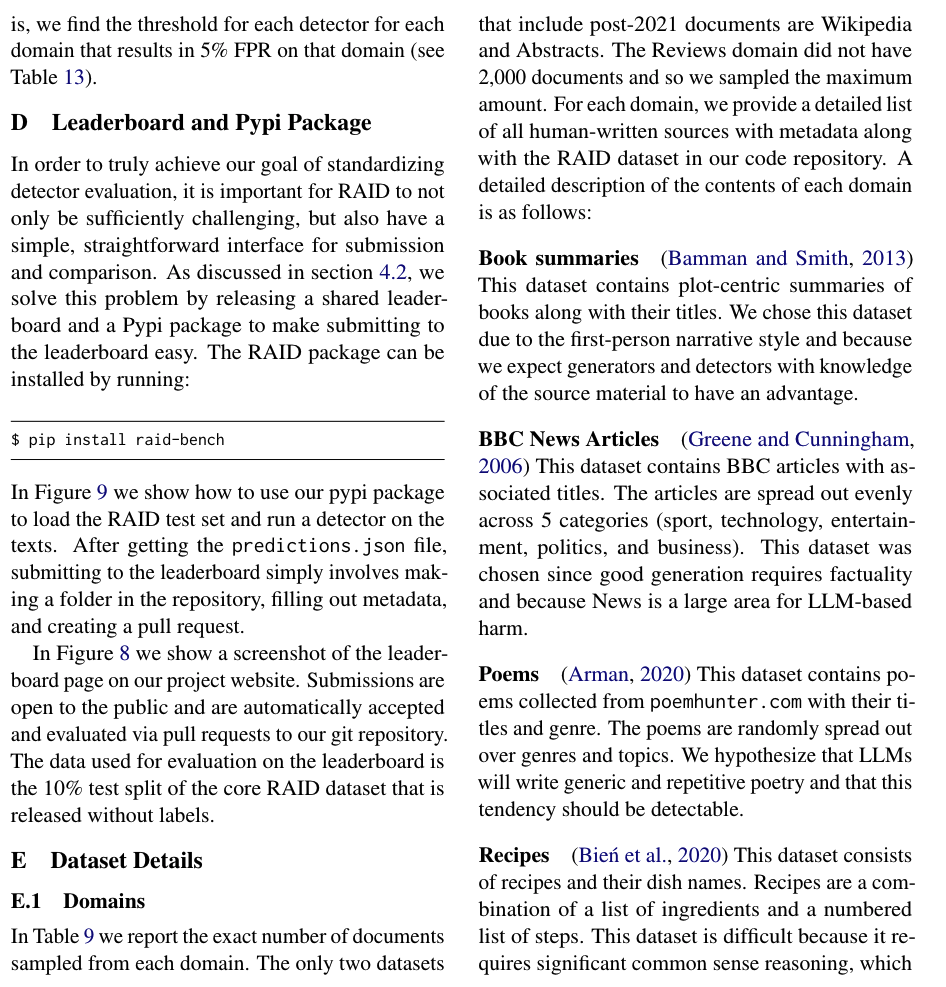
For section E, it describes these dataset details and then states what the expection for the generatons and detectors have. Like for poems, that LLMs will generate repetitive poetry and the detectors can actually detect them. or that recipes are a difficult dataset. It’s suprising how llms counter our intuition. I wonder why that is.
The results conflict, interesting. Shown below in figure 12.
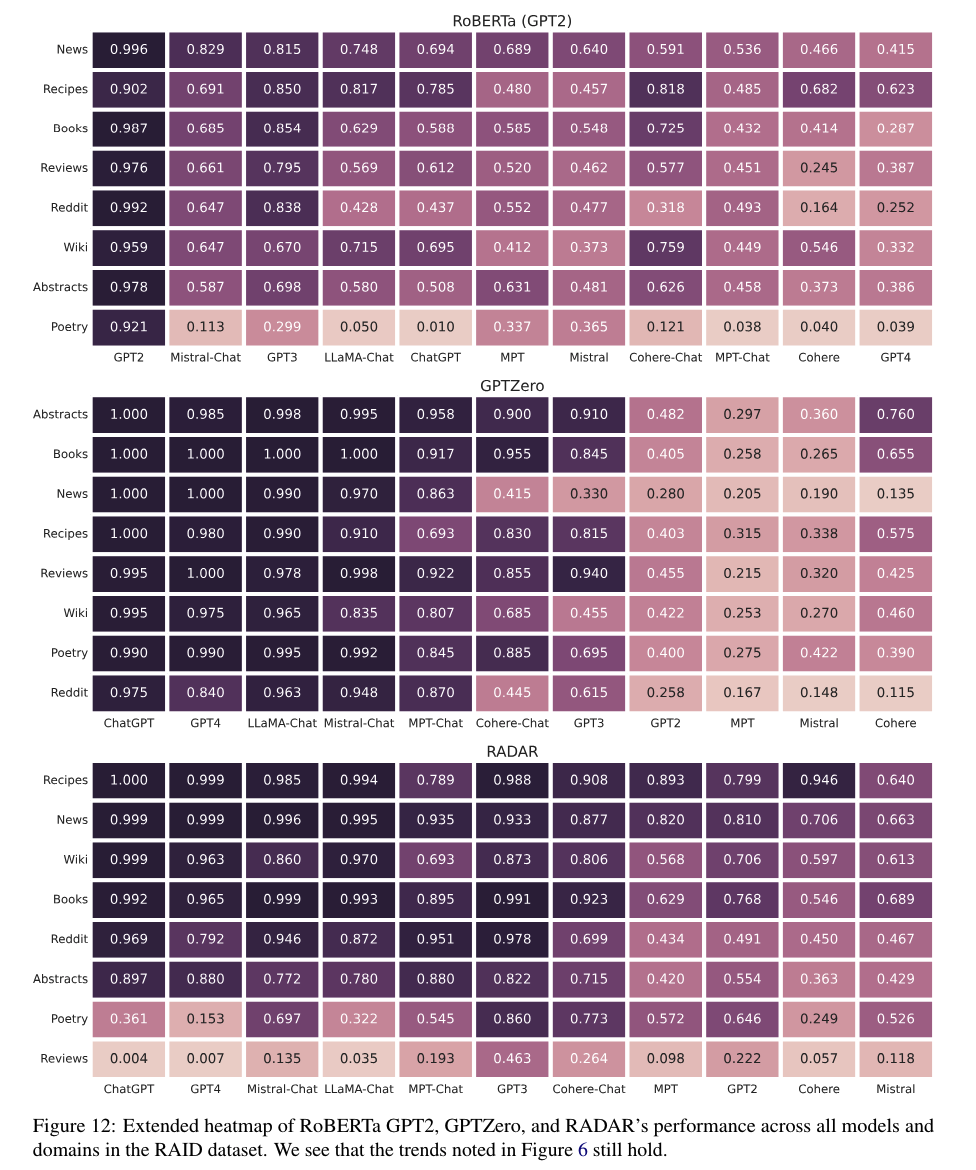
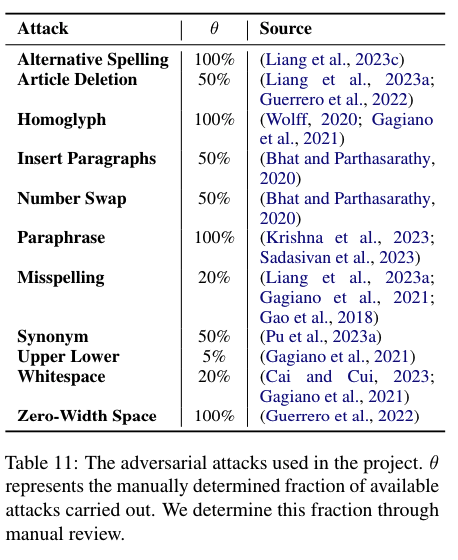
Why this fraction through manual review?
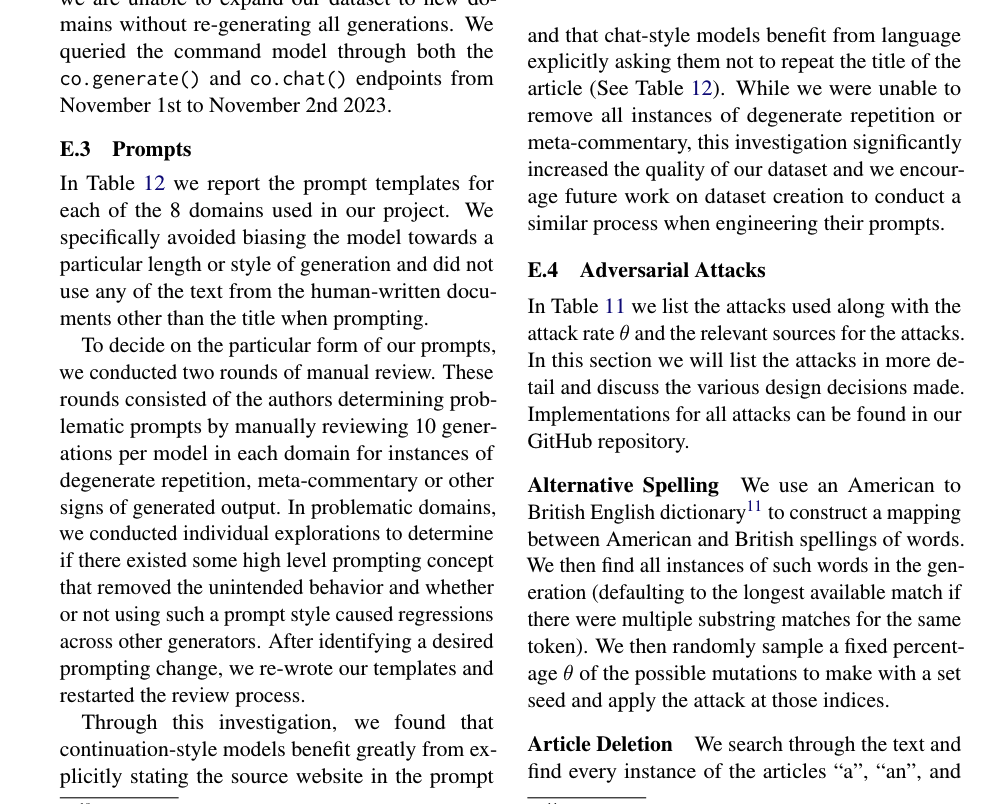
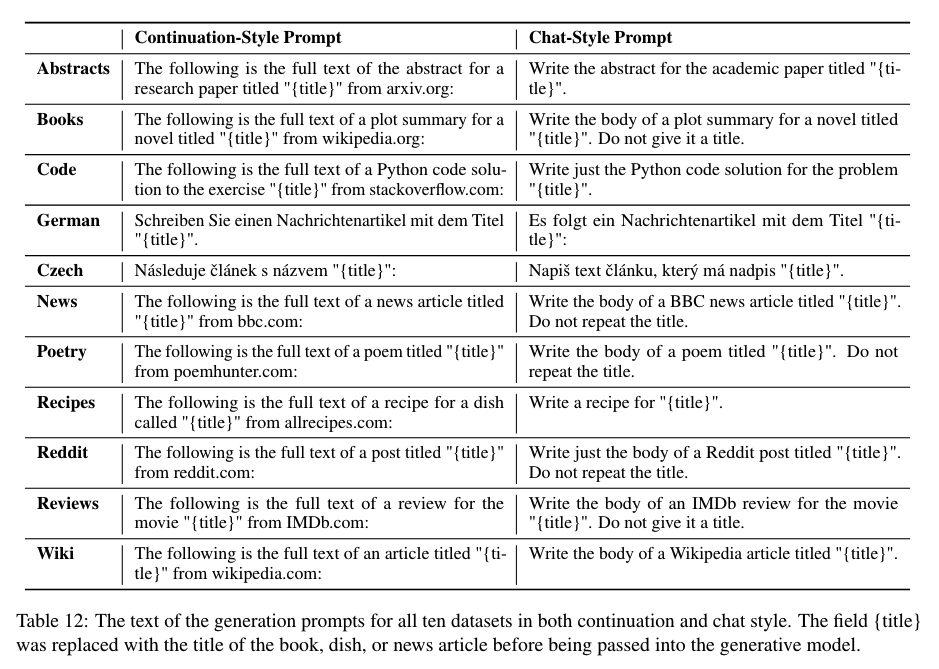
I wonder what the manual review process is to get to that point. What worked and what didn’t? And what would prompt engineers do better? (I don’t know anything about prompt engineers.) And what is happening in the model that makes it better with these prompts. Is there a debugging way like you do with programs (most likely not). Why not use dSpy?
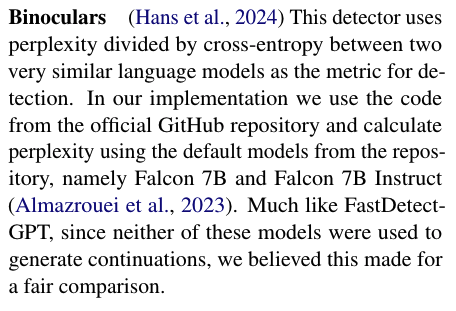
The one I found cool is binoculars. What does this even mean? Perplexity divided by cross-entropy between two llms.
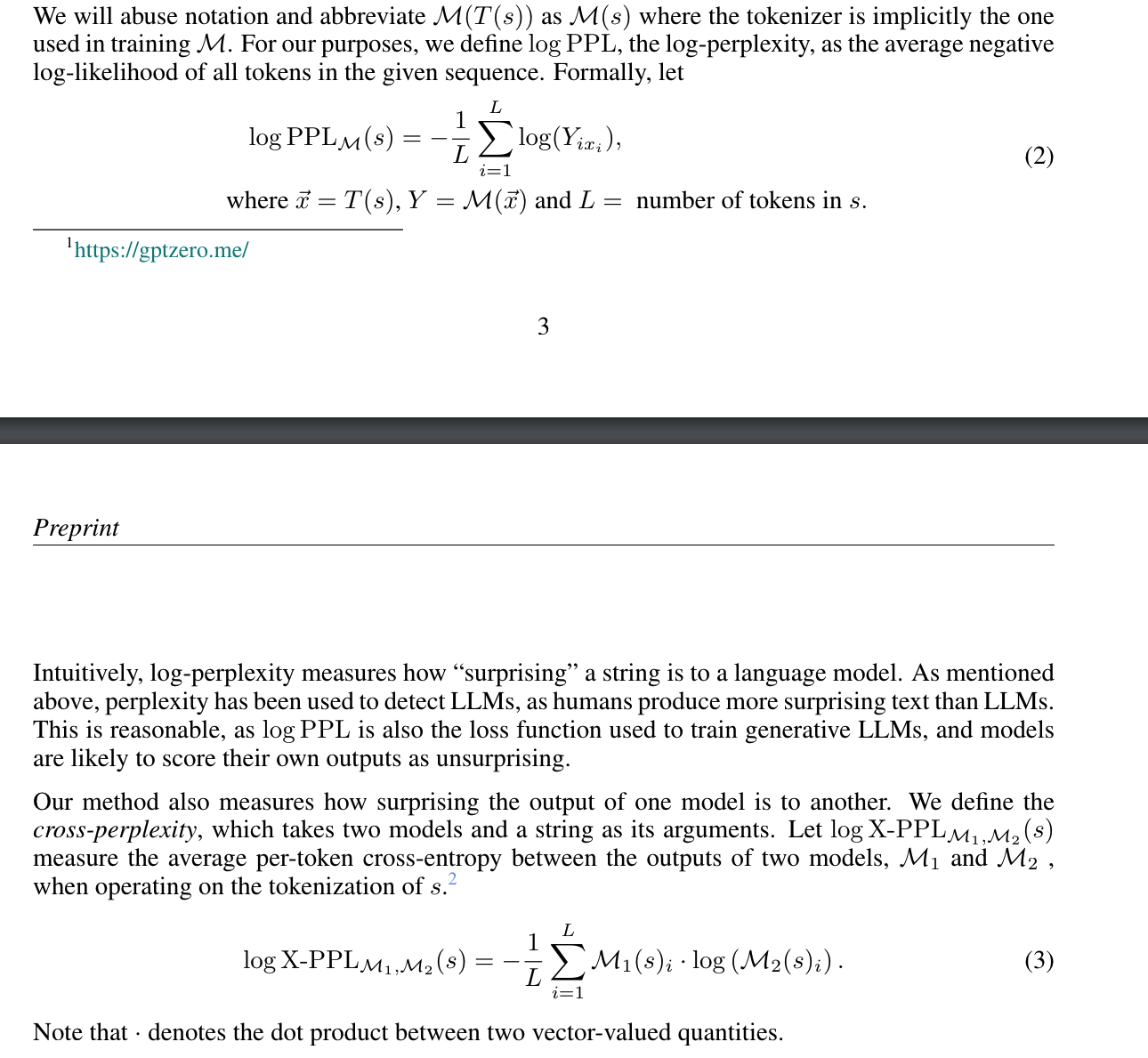
So this is how surprising a text from one model to another. Interesting. I have no idea what this means.
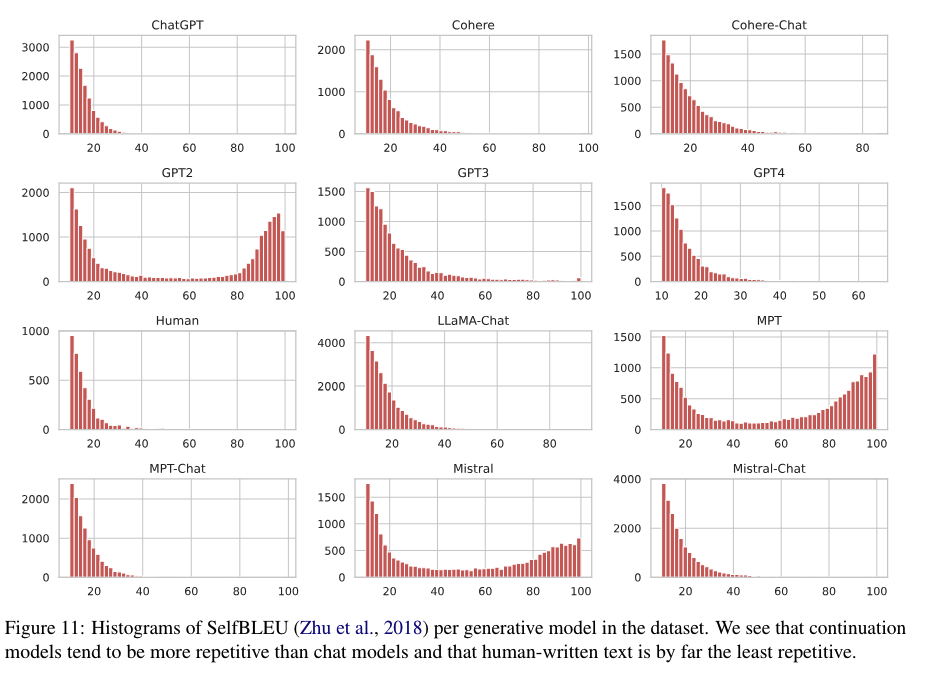
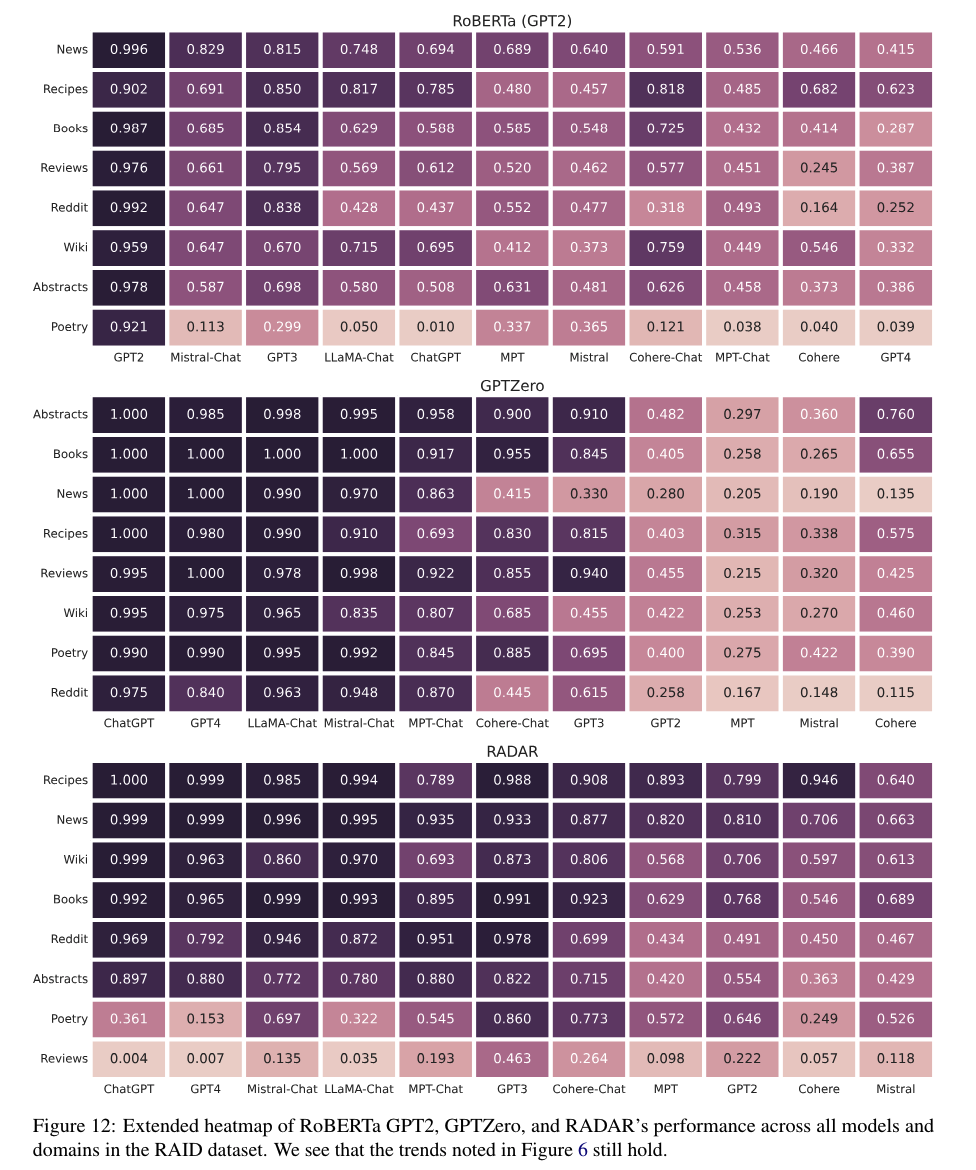
I would say fig11 and fig12 are the most interesting parts of the paper. Fig11 show the histogram of perplexity per model. Why is the human one normal distribution like? It would be interesting to see the examples of each text of the human versus model at X perplexity. Almost none of the models match it. Could we just build a model classifier based on some perplexity metric? (like guess the perplexity) because there are just only some models out there really…
For Fig 12, It’s so weird that ChatGPT and MPT-Chat has the same-ish Self-BLEU. It doesn’t match Table 3, for ChatGPT and mistral. I guess the histogram definitely shows more details.
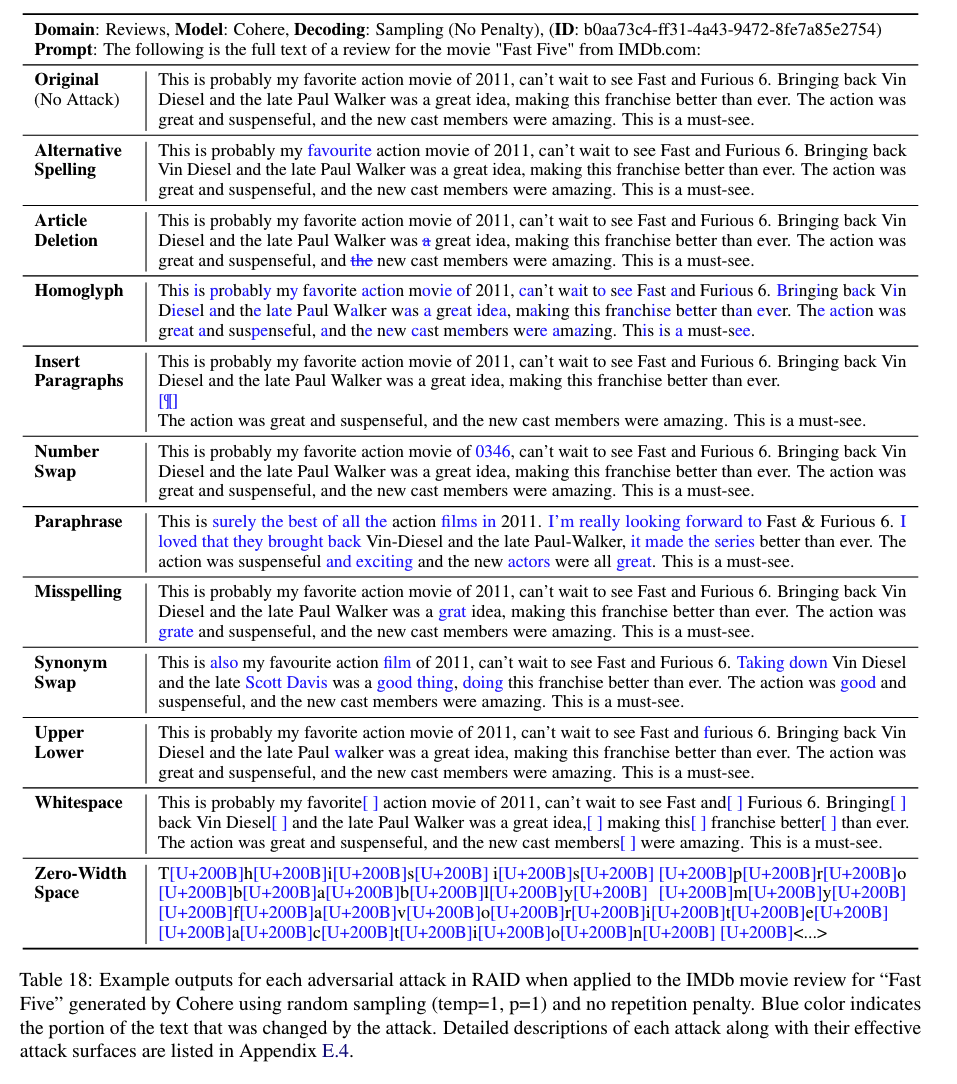
Dude I needed this eariler.
Leaderboard
Yeah, leaderboard works and is super fun to play with. Lots of work put into this! Nitpicking me ~ Would like an example for each of generation with example of attack.
Conclusion
Super nice & impressive paper, has a lot of details and easy to read (esp, since I don’t have a ML/NLP background). Each model/dataset/attack is explained as simply as possible. Graphs/Tables were all explained clearly. It’s very very good. I wonder what inspiration/motivation drew to this type of paper?
Ideas to improve
Please put this out on reddit/hackernews/whatever.
To encourage people to add their own detectors, yeah I have no clue. Maybe like talk to them about their detector or something?
This reminds me of fuzzing blackbox programs. Maybe some notion of coverage and feedback loop can be introduced to build a specific model/program to detect ml-generated text. So. for example, generate a corpus of say shakespeare. Start manipulating the corupus (using the adv attacks) and see how the model responds. Use perplexity or self-bleu or acc or combination to eval. If it gets above/below some metric, mark it as ml generated. Then take a look and start to figure out what manipulations caused the model to show that it’s a model generating the text. It should produce some very interesting results (I hope).
Enjoy Reading This Article?
Here are some more articles you might like to read next: